Leaderboards
Contents
The Spoken Term Discovery task is still very challenging and has not received the same attention as Acoustic Unit Discovery. One major finding across the three ZRC editions that featured this task is the existence of a tradeoff between attempting to find a lot of words and ensuring that the discovered words are accurate. The quality of the set of words that are treated as matches/repetitions by the system, as measured by the normalized edit distance (NED), will necessarily be better if systems do not commit to extracting more dubious word candidates in the first place; however, the more candidates are ignored, the less of the corpus will receive an analysis (lower coverage) and the fewer of the gold word boundaries will be found (leading also to lower boundary F-scores). The tradeoff between term quality and coverage is shown in Figure 3.
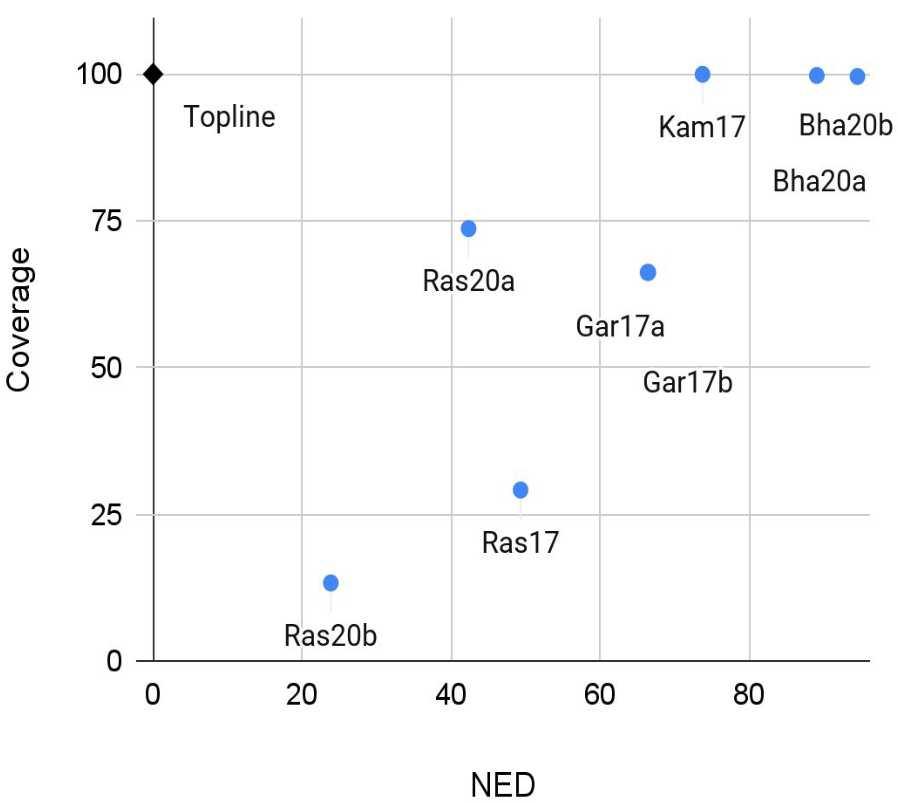
Fig. 3. NED vs Coverage Tradeoff. Average results across 5 languages of TDE-15 (ZR17 and ZR20).
Figure 4 focuses on the segmentation task and displays the Token F-score for each of submitted systems, compared to a topline unigram adaptor grammar segmentation system trained on the corresponding text (phonemized text without the blank spaces between words). All the high-coverage segmentation-oriented models are on the left and all the low NED, matching-first models on the right. The segmentation-oriented models are more likely to do well on this metric, which assesses how many of the true word tokens were correctly segmented. Included here are two new models Kam22 and Alg22 which do not even attempt building a lexicon of types.
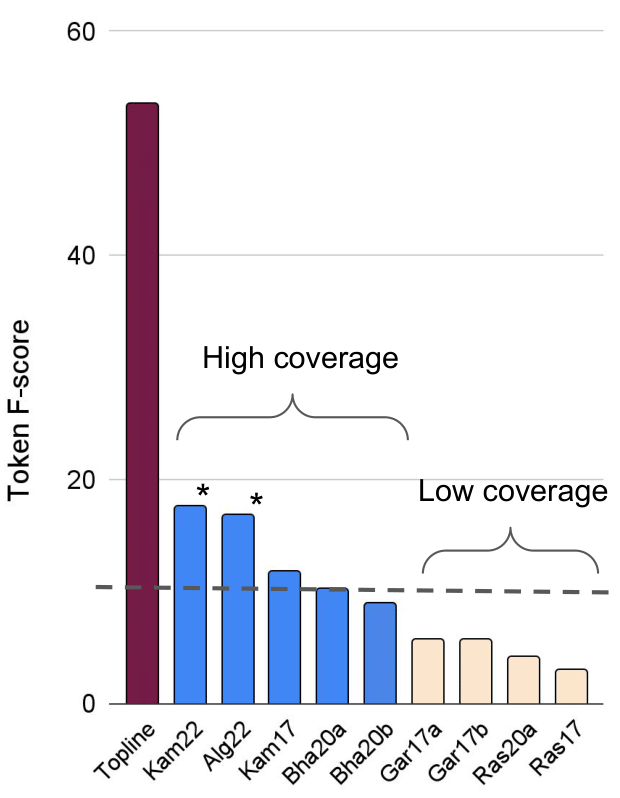
Fig. 4. Token F-scores, measuring how many words are correctly segmented, averaged across 5 languages (ZR17 and ZR20 plus two new papers). The topline is a unigram word segmentation adaptor grammar trained on the same amount of text. The dotted line is a baseline consisting in random segmentations every 120ms. Starred (*) models compute the segmentation without building any lexicon of discrete types.
TDE-15 Leaderboard
English | Xitsonga | ||||||||
---|---|---|---|---|---|---|---|---|---|
# | Author | Model ID | NED | COV | Words | NED | COV | Words |
TDE-17 Leaderboard
English | French | Mandarin | German | Wolof | ||||||||||||||
---|---|---|---|---|---|---|---|---|---|---|---|---|---|---|---|---|---|---|
# | Author | Model ID | NED | COV | Words | NED | COV | Words | NED | COV | Words | NED | COV | Words | NED | COV | Words |